The Future of Precision Farming: The Role of AI and Data Analytics
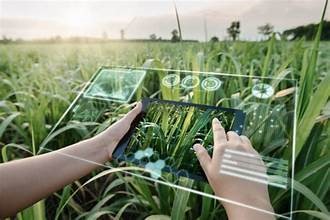
Introduction
Synopsis of Precision Agriculture
Precision farming, sometimes referred to as precision agriculture, is the application of cutting-edge technologies and data-driven methods to improve the sustainability and productivity of agriculture. This novel strategy monitors and controls field variability in order to maximize agricultural yields, reduce waste, and enhance resource efficiency. Farmers can collect and evaluate data on soil conditions, crop health, weather patterns, and other topics by utilizing technologies like GPS, remote sensing, and Internet of Things devices. This allows for better decision-making and focused interventions.
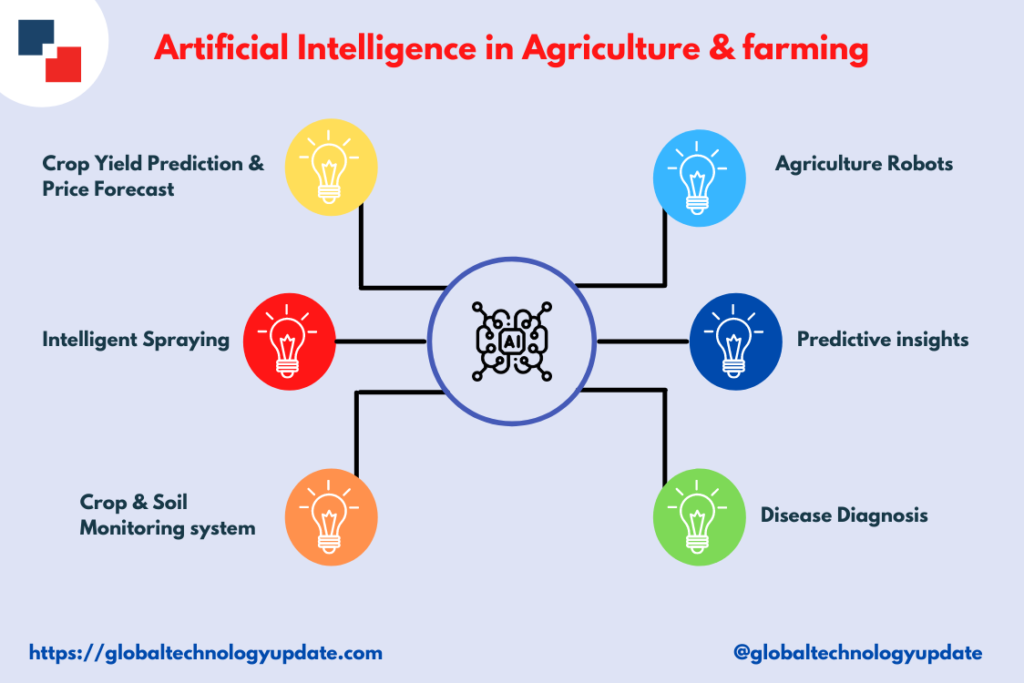
Importance of Technology in Modern Agriculture
The demand for food is predicted to increase dramatically as the world’s population grows, presenting a serious threat to conventional farming methods. Technology’s ability to boost productivity and lessen its negative effects on the environment is vital in tackling these issues. Precision farming is changing as a result of artificial intelligence (AI) and data analytics, which allow farmers to use massive amounts of data to make decisions in real time that improve productivity and support sustainable practices.
Through the integration of these technologies, farmers can attain a more accurate comprehension of their farming systems, which can ultimately result in enhanced yields, less resource usage, and increased resilience against market swings and climate change. Precision farming will become the norm when artificial intelligence and data analytics are used with efficiency farming is essential to the creation of sustainable food, not just a fad.
Agri Innovation and Technology demonstrated in Bharat Agri Tech 2025, an Central India’s Leading Agriculture Exhibition Held in Indore.
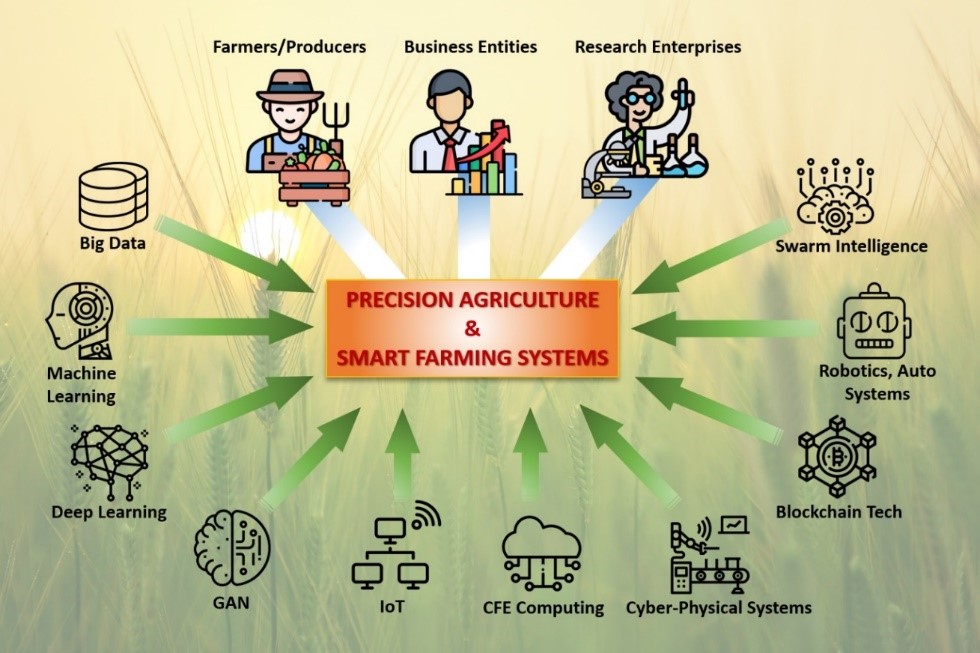
Technology’s Role in Contemporary Agriculture
The production of food is changing as a result of the incorporation of technology into contemporary agriculture, becoming more robust, sustainable, and efficient. Several significant elements demonstrate the significance of technology in modern agricultural practices as we examine the future of precision farming, especially through the lenses of artificial intelligence and data analytics.
1. Improved Decision-Making
Data-Driven Insights:
Farmers can get a tonne of information about crop performance, weather trends, and soil health. By analyzing this data and producing useful insights, AI systems can help decision-makers make better use of resources and increase crop yields.
Predictive analytics: By using real-time data and historical data, predictive models can forecast disease incidence, pest outbreaks, and the best times to harvest, enabling farmers to take preventative action.
2. Enhanced Productivity
Automation of Processes: By streamlining operations and cutting human costs and time, technologies like robotic harvesters, self-driving tractors, and drones simplify operations. Precision automation can execute monotonous duties, freeing farmers to concentrate on more advanced management.
Precision Application: By enabling the focused application of inputs like insecticides and fertilizers, variable rate technology minimizes waste and its negative effects on the environment.
3. Managing Resources
Water Conservation: In areas where water is scarce, smart irrigation systems that make use of soil moisture sensors and weather forecasts to maximize water consumption. This improves crop resilience while simultaneously conserving resources.
Nutrient optimization: Farmers can apply the appropriate amount of nutrients at the appropriate time to improve soil health and crop quality while cutting expenses with the use of sophisticated soil mapping and analytics.
4. Environmental Impact and Sustainability
Decreased Chemical Usage: Technology can contribute to a reduction in the need for chemicals by applying inputs more precisely, improving ecosystem health and lowering pollutants.
Preservation of Biodiversity: Crop rotation and cover crops are two techniques that precision farming promotes and which improve soil health and biodiversity, leading to a more sustainable agricultural system.
5. Competitiveness in the Market
Better Market Access: Technology makes it easier to trace supply chains and increases transparency, which enables farmers to better satisfy market demands and establish direct connections with customers Global Collaboration: Farmers all over the world may share knowledge and work together thanks to digital platforms, which promotes innovation and best practices in agricultural production
The Role of AI in Precision Farming
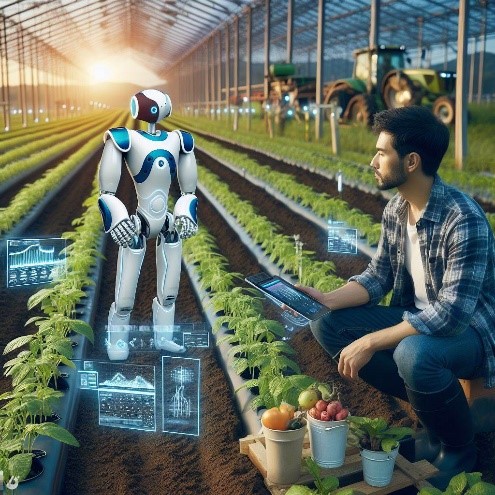
Definition and Explanation of AI:
Artificial Intelligence (AI) is the term used to describe how technology, especially computer systems, can simulate human intelligence processes. Learning (the process of acquiring knowledge and applying rules to it), reasoning (using rules to arrive at approximations or conclusions), and self-correction are some examples of these processes. Artificial Intelligence (AI) in the context of precision farming refers to a collection of technologies that let machines evaluate data, spot trends, and make choices that improve agricultural sustainability and productivity.
Applications of AI in Agriculture
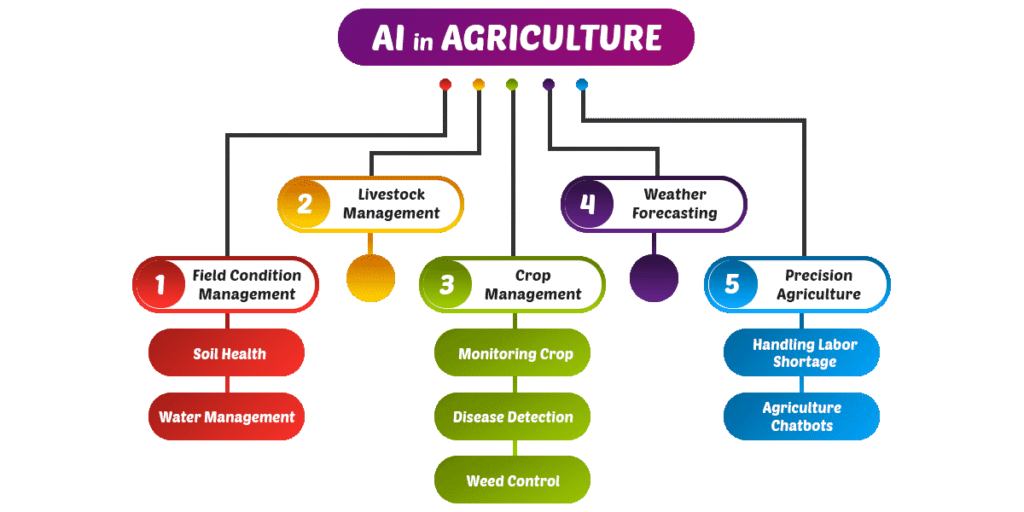
1. Crop Monitoring using Image Recognition
Artificial intelligence (AI)-powered image identification technology is essential to modern agriculture, especially for crop monitoring and health evaluation. Drones, satellites, and ground-based cameras allow farmers to get detailed pictures of their fields. This is how it operates:
– Early Issue Detection: Artificial intelligence systems are capable of analyzing photos to spot crop illnesses, pests, or nutrient deficits in their early stages. Farmers may take action before problems worsen thanks to this prompt diagnosis, perhaps minimizing crop loss.
– Assessment of Crop Health: By examining the reflectance of light at various wavelengths, AI can assess the health of plants through methods such as spectral imaging. With the aid of these data, crop vitality may be assessed generally, allowing for more focused actions.
– Field Mapping and Analysis: AI uses picture processing to produce intricate maps that show how crop health varies throughout fields. Farmers can better manage resources and comprehend spatial heterogeneity with the aid of these insights.
– Automated Reporting: By using picture analysis, AI systems can create automated reports that give farmers clear-cut information on crop conditions, enabling them to make well-informed decisions.
2. Predictive Analytics using Machine Learning
Without explicit programming, machines may learn from data and gradually improve their predictions thanks to machine learning, a subset of artificial intelligence. Machine learning is used in agriculture in a few significant ways:
– Yield Prediction: Machine learning models can accurately forecast crop yields in the future by examining past data, weather trends, and soil characteristics. Farmers can better plan their planting plans and allocate resources by using this information.
– Pest and Disease Forecasting: To predict future pest infestations and disease outbreaks, machine learning algorithms examine data from a variety of sources, including previous pest outbreaks, climatic variables, and crop kinds. Farmers can put preventive measures in place thanks to this proactive approach.
– Irrigation Management: Using previous irrigation data and weather projections, machine learning models can estimate soil moisture levels and water requirements. This makes it possible to plan irrigation schedules that maximize crop moisture retention while wasting less water.
– Supply Chain Optimization: To forecast product needs and optimize supply chains, machine learning is used to analyze logistical data, consumer demand, and market trends. This guarantees that farmers can more effectively match output to market demands.
Data Analytics in Agriculture
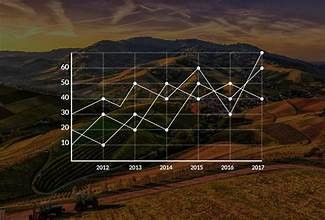
1. Definition of Data Analytics
The methodical computational examination of data sets to find patterns, trends, and insights is known as data analytics. In order to make well-informed judgments about farming operations, data analytics in agriculture entails processing vast volumes of information received from numerous sources. Farmers can gain important insights that improve productivity and sustainability by applying statistical techniques and machine learning algorithms.
2. Data Types Employed
Various kinds of data are gathered and examined in precision farming, such as:
Satellite Photography: Large agricultural fields can be seen from above with the use of satellite pictures. They provide insightful data on soil conditions, land use, and crop health. Farmers are able to track crop development and identify stress factors with the aid of sophisticated remote sensing tools that may spot fluctuations in vegetation indices.
Sensor Information:
Real-time data on soil moisture, temperature, nutrient levels, and meteorological variables are gathered by sensors positioned on the ground. Effective management of pest control, fertilization, and irrigation depends on this data. Because IoT devices can communicate data continuously, farming techniques may be dynamically adjusted.
Historical Information on Yield:
Past performance indicators for various crops under various conditions make up historical yield data. By examining this data, trends and patterns can be found that can guide planting choices in the future as well as crop rotation plans and risk management techniques.
3. The Value of Gathering and Analyzing Data
Agriculture needs data gathering and analysis for a number of reasons.
Well-Informed Decision-Making: Farmers are better equipped to decide on planting, resource allocation, and risk management when they have fast and accurate access to data. As a result, agricultural yields are increased and waste is decreased.
Resource optimization: Data analysis makes it possible to apply inputs like herbicides, fertilizers, and water precisely. By lowering resource overuse, this decreases environmental effect in addition to saving money.
Risk Mitigation: Farmers can foresee difficulties like weather variations, pest outbreaks, or shifts in the market by employing predictive analytics. Better planning is made possible by this proactive approach, which also lowers potential losses.
Increased Productivity and Sustainability: A deeper understanding of agricultural systems is fostered by data analytics, which results in more effective practices. This promotes environmentally friendly, sustainable farming practices while also increasing productivity.
Benefits of AI and Data Analytics in Precision Farming
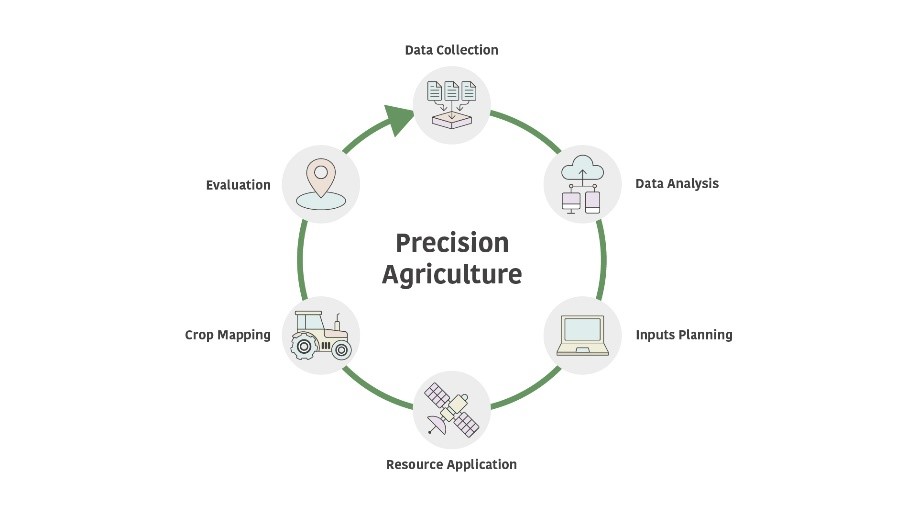
Data-Based Perspectives
Data-driven insights from AI and data analytics are essential to precision farming because they change agricultural practices. With the help of these information, farmers may make decisions that improve efficiency, sustainability, and productivity.
1. Real-time Soil and Crop Monitoring
One of the biggest benefits of artificial intelligence and data analytics in agriculture is the ability to monitor crops and soil in real time. This is how it operates:
Continuous Data Collection: Temperature, pH, nutrient content, and soil moisture are all measured in real time by Internet of Things (IoT) sensors positioned in fields. Through ongoing observation, farmers are able to comprehend the current circumstances influencing their crops.
Crop Health Assessment: High-resolution photographs of fields can be taken by drones fitted with cutting-edge imaging technology, enabling a thorough examination of plant health. These photos are processed by AI algorithms that detect illness, insect infestations, or stress signals, allowing for prompt intervention.
Irrigation Management: Farmers can optimize irrigation schedules and make sure crops receive the appropriate quantity of water when they need it by using real-time data on soil moisture levels. This encourages healthy development while simultaneously conserving water.
Yield Prediction: Farmers may more accurately estimate yields, enabling improved planning and resource allocation, by combining historical trends with real-time data analysis.
2. Case Studies Highlighting Effective Implementations
A number of case studies demonstrate how AI and data analytics are successfully used in precision farming:
Example 1: Climate Corporation
A division of Bayer, The Climate Corporation leverages AI and machine learning to give farmers insights from massive data analysis. Their technology provides personalized planting and resource management suggestions by analyzing historical yield data, weather patterns, and soil conditions. Increased yields and lower input costs have been reported by farmers utilizing this technology.
Example 2: John Deere
John Deere’s precision farming equipment incorporates artificial intelligence and data analytics. The company’s equipment is fitted with sensors that gather information on the state of the field during planting and harvesting. To increase operational efficiency and optimize machine settings, this data is evaluated. Farmers that use John Deere technology have seen notable increases in output and decreases in waste.
Example 3: Ecorobotix
An autonomous weed-eating robot made by Ecorobotix employs artificial intelligence (AI) to detect and locate weeds while using fewer herbicides. The robot uses machine learning to discern between crops and weeds while taking pictures of crops in real time. For farmers who have embraced this technology, precision application has enhanced crop health and resulted in a significant decrease in pesticide use
Analytics for Predictive
Farmers can now predict future conditions and events thanks to predictive analytics, a potent combination of artificial intelligence and data analytics. This insight improves resource management and decision-making, which eventually results in more productive farming methods.
1. Forecasting Pest Outbreaks and Weather Patterns
Weather forecasting: Artificial intelligence (AI) algorithms examine enormous datasets, such as satellite imagery, historical weather data, and current meteorological information. By offering precise weather projections for both the short and long term, these models help farmers arrange their planting, irrigation, and harvesting schedules more efficiently.
Prediction of insect and Disease: Machine learning algorithms are able to recognize patterns that indicate the likelihood of insect outbreaks and the transmission of diseases by examining past data on pest populations, crop health, and environmental factors. Farmers can reduce possible damage by implementing preventative measures, such as targeted pesticide applications or crop rotations, by identifying these patterns.
Integrated Risk Management: Farmers can create all-encompassing risk management plans by combining weather and pest forecasts. Farmers should take preventive measures to safeguard their crops, for example, if a weather forecast indicates more humidity, which is conducive to specific pests.
2. Instruments and Systems Employed
Predictive analytics is used in a number of precision agricultural techniques and platforms, including:
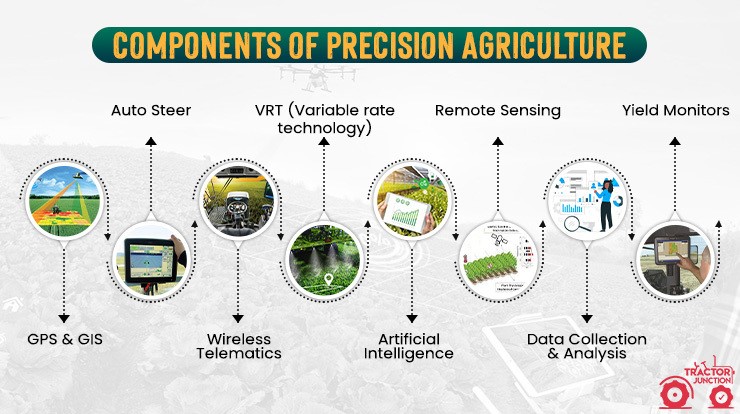
Climate Field View: With the use of localized predictions and real-time meteorological data, this tool assists farmers in making well-informed decisions. It combines field data and satellite pictures to offer insights into crop performance and environmental circumstances.
IBM Watson Decision Platform for Agriculture: This all-inclusive platform analyzes data from several sources, such as pest reports and weather forecasts, using artificial intelligence. It offers farmers practical knowledge to enhance crop resilience and maximize resource utilization.
CropX: This soil sensing device evaluates the amount of nutrients and moisture in the soil, then uses predictive analytics to determine the best times to water. It aids farmers in water conservation and improves crop health by forecasting how weather patterns would impact soil conditions.
AgriWebb: A software for managing farms that makes use of data analytics to predict insect outbreaks and offer management advice for livestock. By anticipating obstacles, its predictive powers assist farmers in optimizing their operations.
Resource Allocation
One of the main advantages of incorporating AI and data analytics into precision farming is resource optimization. Farmers may greatly increase the efficiency of their inputs, resulting in increased productivity and sustainability, by utilizing these technologies.
1. Effective Use of Pesticides, Fertilizers, and Water
– Precision Irrigation: AI-driven systems use weather forecasts and real-time data from soil moisture sensors to pinpoint the precise amount of water that crops require. By focusing on specific areas, this method reduces water waste and guarantees that plants get the proper quantity of moisture when they need it, encouraging better growth.
– Variable Rate Application (VRA): Data analytics makes it possible to apply pesticides and fertilizers precisely according to the needs of various sections of a field. Farmers can minimize unnecessary use and expenses by applying the proper amount of nutrients or chemicals where needed by evaluating soil health data and crop requirements.
– Automated Monitoring Systems: Drones and intelligent sensors keep an eye on field conditions around-the-clock, alerting farmers to pest infestations or nutrient shortfalls. This makes it possible for prompt interventions to focus on particular issues rather of using general inputs.
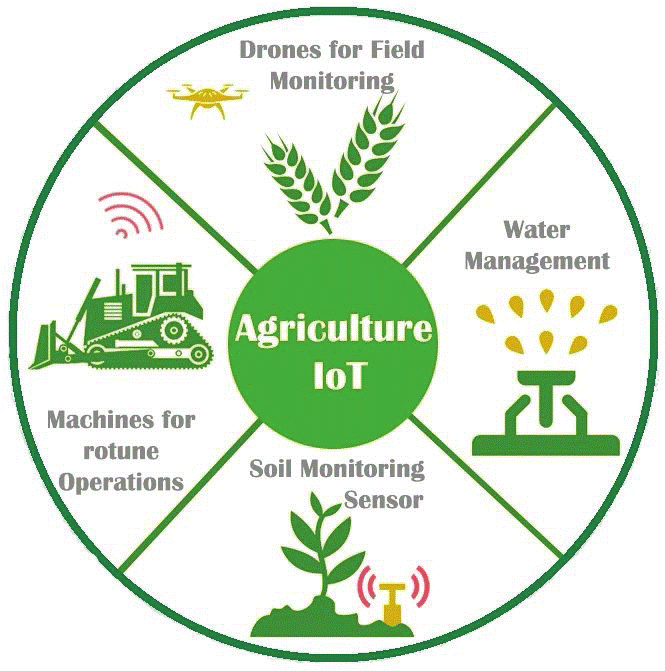
2. Mitigation of Environmental Effects
– Minimized Chemical Runoff: Precision farming techniques assist lessen chemical runoff into surrounding water sources by using pesticides and fertilizers only where needed. This preserves aquatic ecosystems and improves soil health in the long run.
– Improving Soil Health: Using resources wisely helps to promote soil health. Farmers may promote a more resilient agricultural ecosystem by improving soil structure and biodiversity, as well as avoiding overfertilization and preserving adequate nutrient levels.
– Reduction of Carbon Footprint: Reducing greenhouse gas emissions from agricultural activities is achieved through optimizing resource usage. Farmers can lower the energy consumption associated with the production, transportation, and use of these resources by lowering the quantity of inputs required.
- Automated Agriculture Machinery
AI and data analytics-driven automated farming equipment is a major breakthrough in precision agriculture. This technology transforms conventional farming methods by increasing productivity and operational efficiency.
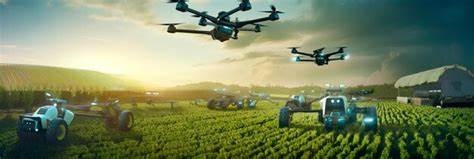
1. Synopsis of AI-Powered Equipment
Artificial Intelligence (AI)-driven machinery comprises a variety of automated devices intended to carry out diverse agricultural operations with minimal human involvement. Important instances consist of:
– Autonomous Tractors: These tractors can navigate fields, plant seeds, and apply fertilizer on their own thanks to GPS, sensors, and AI algorithms. They may work continuously, which boosts productivity.
– Drones: Drones with AI and image capabilities assess crop health, track field conditions, and even help with fertilizer and pesticide application that is targeted. Their aerial viewpoint offers insightful information that is not possible with ground-based devices.
– Robotic Harvesters: These devices recognize ripe fruits and vegetables using artificial intelligence, enabling accurate harvesting. They can perform tasks more reliably and quickly than human labor, which lowers labor expenses and lessens agricultural damage.
– Smart Irrigation Systems: AI and sensors are used by automated irrigation systems to decide when and how much water to apply, giving crops the ideal quantity of moisture without wasting any.
2. Advantages of Automation in Agriculture Methods
There are several advantages of integrating automated farming equipment:
– Enhanced Efficiency: Because automated equipment may run constantly, downtime is decreased and productivity rises. Planting and harvesting, two tasks that formerly required a lot of effort, may now be completed more swiftly and effectively.
– Cost Reduction: Automation can save farmers a lot of money by reducing labor expenses and maximizing the use of resources (such as water and fertilizers). Higher profitability is made possible by this economic efficiency.
– Enhanced Precision: AI-driven equipment completes tasks with extreme precision, lowering the possibility of human error. Better yields and healthier plants result from improved crop management brought about by this precision.
– Labor Shortages Mitigation: Particularly during peak seasons, the agriculture industry frequently experiences a labor shortage. By filling up these gaps, automation makes sure that crucial tasks are finished on schedule.
– Sustainability: Sustainable agricultural methods benefit from the more effective use of resources that automated systems provide. Lowering input consumption helps farmers achieve sustainability goals by reducing their impact on the environment.
Forecasted Yield
A key component of precision farming is yield prediction, which uses AI and data analytics to predict crop performance with accuracy. Advanced yield forecasting tools enable farmers to make well-informed decisions that improve productivity and profitability.
1.Methods for Predicting Yields
Models for Machine Learning: These algorithms examine past yield data in addition to meteorological trends, soil quality, and crop genetics. Machine learning algorithms are highly accurate in forecasting future yields by identifying intricate linkages within the data.
Satellite imagery and remote sensing: State-of-the-art imaging techniques offer data on crop health and development phases in real time. Farmers can determine growth patterns and anticipate yields prior to harvest by examining these photos.
Weather Data Integration: Farmers can take into account climatic factors that affect crop growth by combining forecast models with historical weather data. Regression analysis is one technique that aids in assessing the impact of various weather conditions on yields.
Soil Analysis: Yield prediction depends heavily on the condition of the soil and its nutrient levels. In order to improve accuracy, real-time data from advanced soil sensors can be included into prediction models.
Crop Simulation Models: These models replicate growth circumstances according to a range of parameters, including farming practices, soil composition, and weather. They aid in yield forecasting under various conditions, enabling farmers to make appropriate planning decisions.
2. Farmers’ Economic Implications
For farmers, accurate yield prediction has major financial ramifications:
Better Planning and Resource Allocation: Farmers may more effectively plan their planting schedules, irrigation requirements, and input applications by using yield predictions. By optimizing, waste is decreased and overall efficiency is improved.
Market Strategy Development: Farmers can decide how best to market their produce by having a clear understanding of predicted yields. They can maximize revenues by timing sales to align with advantageous market conditions.
Risk management: By forecasting yields, producers may prepare for probable deficits and put risk-reduction plans in place, like crop insurance or crop variety diversification. This proactive strategy aids in income stabilization.
Making informed judgments about investments in new technology, machinery, or crop varieties is aided by accurate forecasts. Based on anticipated results, farmers can rank the investments that will provide the highest returns.
Financial Planning: Farmers can better manage cash flow and financial planning, which will result in more stable operations and the capacity to pay expenses during hard times, by projecting returns ahead of time.
Efficiency of the Supply Chain
Modern agriculture depends heavily on efficient supply chains, where artificial intelligence (AI) and data analytics are revolutionizing the industry. Through the optimisation of logistics and the analysis of market demand, these technologies augment the agricultural supply chain’s overall efficiency and efficacy.
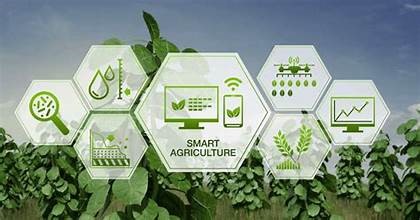
Forecasting demand:
In order to forecast future demand for agricultural products, AI models examine market trends, consumer preferences, and past sales data. This knowledge lowers the possibility of overproduction or spoiling by enabling farmers to match production to market demands.
Farmers are better able to choose crops and plant strategies that will provide high-quality produce by knowing demand trends.
Management of Inventory:
Artificial intelligence (AI)-driven technologies make inventory management easier by forecasting product requirements and quantities. This reduces waste and avoids surplus inventory, which improves resource utilization.
Farmers and distributors can quickly adapt to changes in demand by altering production and supply when inventory levels are tracked in real-time.
Transparency in the supply chain:
Artificial Intelligence (AI) solutions improve supply chain transparency by giving real-time information on product movement and conditions. Stakeholders are better able to make educated decisions and react quickly to problems like delays or quality issues when there is visibility.
By offering unchangeable transaction records and fostering more accountability and trust among supply chain actors, blockchain technology can be used in conjunction with AI.
Tailored Agriculture Methods
Developing personalized farming procedures is one of the major benefits of AI and data analytics in precision farming. Farmers may maximize their operations for higher yields and more efficient use of resources by customizing techniques to particular crops and local conditions.
1. Creating Customized Plans for Various Crops and Areas
Solutions Specific to Crops: Artificial intelligence examines information on certain crop varieties, such as growth habits, nutrient requirements, and insect susceptibilities. This makes it possible to create customized agronomic techniques that optimize each crop’s potential and produce higher-quality and more abundant harvests.
Regional Adaptation: Variations exist in soil composition, insect pressures, and climate conditions among different regions. Farmers are able to evaluate local environmental elements and modify their farming practices based on the insights provided by AI and data analytics. An effective method in a humid climate might not be appropriate in a dry one.
Precision Input Application: Farmers may identify the best times and amounts to apply water and fertilizer by combining data on crop needs and soil health. Crop performance is improved and waste is decreased by this adjustment.
Adaptive Management operations: Farmers may make real-time adjustments to their operations since AI systems are able to continuously learn from new data. Being flexible is essential for reacting to shifting weather patterns and new pest threats.
Systems for Supporting Decisions
With the aid of decision support systems (DSS), farmers are able to make well-informed decisions based on thorough data analysis.
1. Instruments that Help Farmers Make Knowledgeable Decisions
Software for Farm Management: Ag Leader and Trimble are two examples of platforms that offer integrated solutions for gathering, analyzing, and visualizing data from several sources. Farmers may better organize their finances and schedule everything from planting to harvest with the aid of these tools.
Predictive analytics platforms: By utilizing machine learning, these tools are able to predict future circumstances by analyzing historical data. Strategic planning is aided by platforms like as IBM Watson Decision Platform for Agriculture, which provide data on market trends, pest outbreaks, and weather impacts.
Mobile Apps: A lot of decision support tools are now offered as mobile applications, giving farmers on-the-go access to real-time information and insights. This accessibility facilitates prompt decision-making and improves responsiveness to changing circumstances.
Tools for Scenario Analysis: With the help of these tools, farmers can model several management scenarios and assess possible results depending on changing inputs and environmental factors. Farmers can select the best course of action by being aware of the consequences of various approaches.
Obstacles and Things to Think About
Precision farming can greatly benefit from the integration of AI and data analytics, but there are a number of issues and concerns that need to be taken into account in order to make the move successful.
1. Concerns About Data Security and Privacy
– Sensitive Information: A large amount of data, including confidential information regarding farming methods, crop yields, and financial performance, is produced by the agricultural industry. It is imperative to protect the privacy of this sensitive data since breaches may result in a disadvantage over competitors.
– Cybersecurity Risks: Farms are more vulnerable to cyberattacks as they use linked technology (IoT devices, cloud storage). Sustaining trust with farmers and stakeholders requires safeguarding against data breaches and guaranteeing system integrity.
– Regulatory Compliance: If farmers share their data with other parties, such technology companies or academic institutions, they will need to comply with a number of requirements pertaining to data protection. To prevent legal ramifications, it is essential to comprehend and abide by these requirements.
2. The Need for Training and Education for Farmers
– Skill Gaps: The workforce has to be informed about AI, data analytics, and precision farming methods due to the quick progress of technology in agriculture. It’s possible that a lot of farmers lack the technical expertise needed to use these instruments efficiently.
– Training Programs: To guarantee that farmers can properly use AI and data analytics, extensive training programs must be implemented. Workshops, virtual classes, and practical instruction can aid in closing the knowledge gap embrace new methods and technologies. In order to facilitate the transition and promote an innovative culture within the agricultural community, change management measures are essential.
3. First Investment Expenses and Return on Investment Factors
– High Initial Costs: Adopting AI and data analytics technology sometimes necessitates large upfront expenditures for infrastructure, software, and hardware. These expenses may be unaffordable for many farmers, particularly smallholders.
– Return on Investment (ROI): Before implementing precision agricultural technologies, farmers should carefully consider their possible ROI. Even though advantages like higher yields and lower input costs might result in long-term savings, early evaluations can be difficult because these benefits may not be felt right away.
Access to Funding: Obtaining grants or funding to encourage the adoption of new technologies can present challenges. Farmers might want help figuring out where to find money or creating business cases that show their investment is worthwhile.
Prospective Patterns
The field of precision farming is always changing due to new developments in technology and creative methods. A few major themes are influencing how agriculture will develop in the future as the sector adjusts to new difficulties.
1. New Developments in Precision Agriculture Technologies
Advanced Robotics: With autonomous machines able to carry out tasks like planting, harvesting, and weeding, the application of robotics in agriculture is anticipated to grow. These robots can operate reliably and effectively, which lowers labor expenses and boosts output.
– Artificial Intelligence and Machine Learning: As these fields develop, more complex data analysis and decision-making will be possible. Farmers will be able to optimize operations based on real-time data thanks to these technologies’ enhancement of predictive analytics.
– Remote Sensing technology: Drones, sensor technology, and satellite imagery advancements will give more immediate and precise insights on crop health and environmental conditions. Farmers will be able to precisely intervene thanks to this data, enhancing crop management in general.
2. The Internet of Things’ Potential Impact
– Enhanced Connectivity: From weather stations to soil sensors, IoT devices will link more and more components of farming operations. Real-time data collecting and exchange will be made easier by this connectivity, resulting in better decision-making.
Smart Farming Solutions: Internet of Things (IoT)-enabled solutions will give farmers deep insights into their operations, enabling automated management and monitoring. For instance, real-time soil moisture data can be used by intelligent irrigation systems to modify water usage.
– Data-Driven Agriculture: By integrating IoT devices, enormous volumes of data will be produced that can be examined to spot patterns and improve procedures. Farmers will be able to increase crop yields, decrease waste, and improve efficiency with this data-driven approach.
3. Supply Chain Transparency with Blockchain Integration
– Traceability: Agricultural products may be transparently and securely tracked from farm to consumer thanks to blockchain technology. Through the ability to track products’ origins and confirm their legitimacy, stakeholders can improve food safety and quality assurance through traceability.
– Smart Contracts: Supply chain agreements and transactions can be automated by utilizing smart contracts on blockchain systems. This lowers administrative expenses and builds confidence between supply chain participants.
Consumer Trust: Blockchain can offer the transparency required to foster trust as customers’ concerns about the sustainability and provenance of food grow. Farmers may attract a rising market segment that appreciates openness by showcasing their ethical sourcing and environmental practices.
Conclusion
1. Key Points Synopsis
Precision farming’s incorporation of AI and data analytics is transforming the industry, offering a host of advantages and resolving important issues. Important details consist of:
– Data-Driven Insights: Farmers may maximize resource consumption and increase yields by using real-time monitoring and predictive analytics to improve decision-making.
– Resource Optimization: Sustainable practices are promoted by the economical use of water, fertilizers, and pesticides, which also lowers waste and lessens environmental impact.
– Automated Farming Equipment: AI-driven machinery lowers labor costs and increases operational efficiency, enabling farmers to adjust to a labor shortage.
– Yield Prediction: By using sophisticated forecasting methods, farmers may increase profitability by making well-informed decisions regarding planting and resource allocation.
Efficiency of the Supply Chain: Artificial intelligence enhances demand forecasting and logistics, guaranteeing prompt product delivery and cutting down on waste.
– Customized Farming Practices: Based on regional variables, farmers can optimize productivity with techniques designed for certain crops and areas.
– Emerging Technologies: Blockchain, IoT, and robotics will revolutionize precision farming by improving operational efficacy, transparency, and connectivity.
2. Precision Farming’s Future Prospects
Precision farming has a promising future that will see a more efficient, technologically sophisticated, and sustainable agricultural environment. Farmers will have unparalleled access to knowledge and tools to improve their practices as AI and data analytics continue to advance.
In this picture, we anticipate:
– Widespread Adoption of Advanced Technologies: To promote efficiency and sustainability in farming operations, a variety of automated systems, IoT gadgets, and AI solutions will become normative.
*Increased Collaboration: Farmers will participate in networks that foster collaboration, exchanging information and insights to improve regional practices and collective knowledge.
– Enhanced Sustainability: Farmers may improve food security and preserve the environment for future generations by utilizing technology to support more sustainable practices.
– Empowered Farmers: Farmers who have more access to resources, information, and training will be better able to make educated decisions, which will increase their independence and resilience in the face of adversity.
In conclusion, precision farming’s future, supported by AI and data analytics, promises to be a revolutionary industry in the agriculture sector that prioritizes sustainability and innovation while meeting the needs of an expanding global population.
References
1. Fountas, S., et al. (2015). Precision Agriculture Technologies Positively Contribute to GHG Emissions Mitigation. Agricultural Systems, 140, 54-67.
2. Wolfert, S., et al. (2017). Big Data in Smart Farming – A Review. Agricultural Systems, 153, 69-80.
3. Zhang, N., et al. (2002). Precision Agriculture – A Worldwide Overview. Computers and Electronics in Agriculture, 36(2-3), 113-132.
4. Kumar, A., & Gupta, S. (2021). Application of AI in Agriculture: A Review. Artificial Intelligence Review, 54(4), 2529-2548.
5. Gonzalez, R., et al. (2020). Blockchain in Agriculture: A Review of the Opportunities and Challenges. Agriculture, 10(5), 127.
6. Wang, M., et al. (2020). The Internet of Things in Agriculture: A Review. Agricultural Systems, 179, 102740.
7. Thompson, G., et al. (2019). The Role of AI in Precision Agriculture: A Review. Precision Agriculture, 20, 849-870.
8. United Nations (2021). World Population Prospects 2021.
9. Food and Agriculture Organization of the United Nations (FAO) (2018). Digital Agriculture: Farmers’ Perspectives.
10. PrecisionAg Alliance (2020). 2020 Precision Agriculture Trends: What’s Next?